A recent Gartner survey says organizations that are working with artificial intelligence (AI) or machine learning (ML) have, on average, four AI/ML projects in place.
The Gartner “AI and ML Development Strategies” study was conducted via an online survey in December 2018 with 106 Gartner Research Circle Members noted that 59% said that they have AI deployed today.
Jim Hare, research vice president at Gartner, acknowledged a substantial acceleration in AI adoption in 2018. “The rising number of AI projects means that organizations may need to reorganize internally to make sure that AI projects are properly staffed and funded. It is a best practice to establish an AI Center of Excellence to distribute skills, obtain funding, set priorities and share best practices in the best possible way,” he added,
Responded expect to add six more projects in the next 12 months, and another 15 within the next three years (see Figure 1). This means that by 2022, those organizations expect to have an average of 35 AI or ML projects in place.
Figure 1: The average number of AI or ML projects deployed
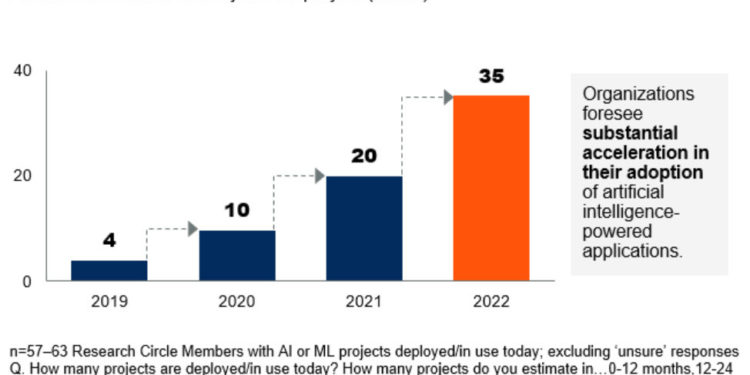
Source: Gartner (JULY 2019)
Drivers
Forty per cent of organizations named customer experience (CX) as their top motivator to use AI technology. While technologies such as chatbots or virtual personal assistants can be used to serve external clients, 56% of the respondent organization today use AI internally to support decision making and give recommendations to employees.
“It is less about replacing human workers and more about augmenting and enabling them to make better decisions faster,” Hare said.
Twenty per cent of respondents named automating tasks as the second most important motivator. Examples of automation include tasks such as invoicing and contract validation in finance or automated screening and robotic interviews in HR.
The top challenges to adopting AI for respondents were a lack of skills (56%), understanding AI use cases (42%), and concerns with data scope or quality (34%). According to Hare, finding the right staff skills is a major concern whenever advanced technologies are involved.
“Skill gaps can be addressed using service providers, partnering with universities, and establishing training programs for existing employees. However, establishing a solid data management foundation is not something that you can improvise. Reliable data quality is critical for delivering accurate insights, building trust and reducing bias. Data readiness must be a top concern for all AI projects,” he explained.
Measuring success
Gartner says many organizations use efficiency as a target success measurement when they seek to measure a project’s merit.
“Using efficiency targets as a way of showing value is more prevalent in organizations who say they are conservative or mainstream in their adoption profiles. Companies who say they’re aggressive in adoption strategies were much more likely instead to say they were seeking improvements in customer engagement,” said Whit Andrews, distinguished vice president, analyst at Gartner.